Ten Ways to Identify High-Quality Physical Climate Data
How to make your way through the competing—and often confusing—claims of the rapidly growing risk data labyrinth
The world of physical climate data is growing ever more complex and bewildering. Climate startups and consultancies are multiplying quickly, offering insights based on a wide range of free and paid data. Users can also download climate model output themselves: for the truly ambitious, the CMIP6 archive of Global Climate Model output has over 2.3 million results to sift through. And, given the global interest in understanding and tackling the greatest challenge of our time, this will only get more labyrinthine over time. How can we make sense of it all? What follows are ten criteria that you should be hunting for to separate the high-quality from the merely mediocre.
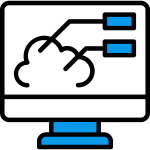
1. State-of-the-art climate models—and the expertise to use them
Climate science moves quickly. Not only has interest in climate science led to more funding and more researchers, but cloud computing has allowed a broader range of scientists to build ever-more-complex models of our world. The most recent CMIP6 generation of climate models, which underpins the newest report from the Intergovernmental Panel on Climate Change, benefits from seven more years of scientific advancements, observations, and emissions data over the earlier, CMIP5 generation. A reliance on outdated models will lead to outdated predictions.

2. A range of independent models, with ensembles where appropriate
As I wrote last fall, using the models from several modeling groups increases the likelihood that the results represent the broader scientific consensus, as opposed to relying on the view from a single group. Furthermore, combining the results of several models makes it easier to pick out the climate signal from other, shorter-term oscillations that appear in climate simulations, like a strong El Niño year.
Acute perils—those with low frequency but high severity, like an extreme rainfall event—require additional care. By definition, they appear rarely: a 1-in-100-year flood event has a 1% chance of appearing in a year-long simulation. To appropriately model these extreme events, scientists need to gather more simulations by using data for the surrounding years (e.g., the year 2040 may be modeled with data from 2035-2045) and using multiple “ensembles” from a model, in which a climate model is run multiple times with slight perturbations to the starting weather conditions. Users should be on the lookout for data that makes judicious use of many climate models, all with ensembles and multi-year spans, for these extreme perils.
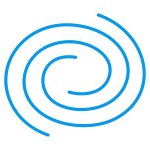
3. Tropical cyclone modeling
Global climate models simply cannot model tropical cyclones, and even if they could, there are not enough simulations of the future to thoroughly blanket the world’s coastlines with all possible future tropical cyclones. Furthermore, we can’t rely on historical data, as that is also not enough “simulations” of what the future may hold. The best solutions combine 1) the cat-modeling technique of using tens of thousands of possible storms, and 2) climate model data for future sea-surface temperatures and other conditions that control the intensities of storms. We can then generate new events for what the future may hold, based on these background climate conditions. This is also an improvement over most “climate-conditioned cat model” techniques, which tend to rely on reshuffling probabilities of the storms that are possible under today’s climate.
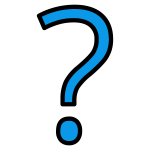
4. Quantified uncertainty
Climate modeling is, of course, quite uncertain, and the magnitude of that uncertainty differs by the peril, the projection year, and other factors. Focusing only on the means is a sure way to be surprised. A responsible climate data provider will communicate standard deviations and/or confidence intervals for all of their metrics, and be clear on the types of uncertainty it incorporates.
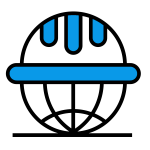
5. Responsible downscaling and machine learning use
Downscaling is the practice of refining coarse climate model outputs to the finer spatial and temporal scales needed to understand weather and other factors that drive local impacts. As Jupiter’s co-founder and chief scientist Dr. Josh Hacker wrote, “a superior downscaling strategy requires flexibility and a toolbox of solutions,” including dynamic, stochastic, and empirical–machine learning (ML) downscaling. A data provider should be able to provide a strong rationale for their choices. Furthermore, if ML is employed, physical scientists still need to be involved to evaluate the resulting models and ensure they are explainable.

6. Verification and validation
A climate data provider should be able to articulate the numerous steps that they’ve undertaken to use valid methods (the methods are sourced from peer-reviewed literature and used appropriately) and verify the outcomes (the data is reasonable: outliers are explainable, there is spatial/temporal consistency, and the data performs well against observations and other models).
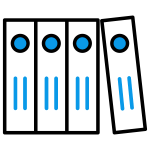
7. Documentation and transparency
Documentation should support a range of ways that the data is being used by a firm. For example, vetting a data provider’s climate scores for use in a TCFD report is less arduous than going through an extensive model risk management (MRM) process before using their flood data to price mortgage risk. Not every data provider is ready for intensive model validation. Even if your first use cases are simplistic, it’s critical to look for a provider that is set up to support you for your long-term climate goals.

8. Decision-grade metrics
There are infinite ways to slice climate data into usable metrics. Even something as simple as rainfall can be tricky: annual precipitation, monthly precipitation, days with significant rain, consecutive dry days, three-day rainfall totals… all are valid ways to think about future precipitation patterns. The most useful metrics are the ones that are useful to you: the ones that most easily map to financial consequences to your firm. Climate data providers should offer a wide range of metrics to lessen the chance that you’ll be forced to accept proxy metrics instead of what really matters to you.

9. Flexible years and scenarios
Just as you require a wide range of climate metrics, those metrics should be provided for a wide range of projection years and scenarios. This allows you to match time horizons to your assets’ remaining useful life, and to match scenarios to whether you need something Paris-aligned, middle-of-the-road, or as a stress test.

10. Transparent scoring methodology
Climate scores are a popular and useful tool to figure out what your climate data is telling you, but they are inherently a judgment call by the data provider. A “good score” and a “bad score” for your assets may be different from a good or bad score for the generic assets that the data provider had in mind. For that reason, it’s important to get the story behind the score: to be able to dive into the scoring methodology and the underlying metrics that drive it, and potentially to create your own scores. Data providers have to support users in this.
‘High-quality climate data in, superior insights out’.
You have many choices when it comes to the climate data you work with, and, unfortunately, a wide range in the quality of that data. Climate analysis is not immune to “garbage in, garbage out”; no matter the skill of the structural engineer, economist, or consultant who is working with the data, low-quality climate metrics will lead to low-quality conclusions. Come prepared with your questions, and be ready to push back if you don’t get the answers you need. And don’t worry—most of us can take it.